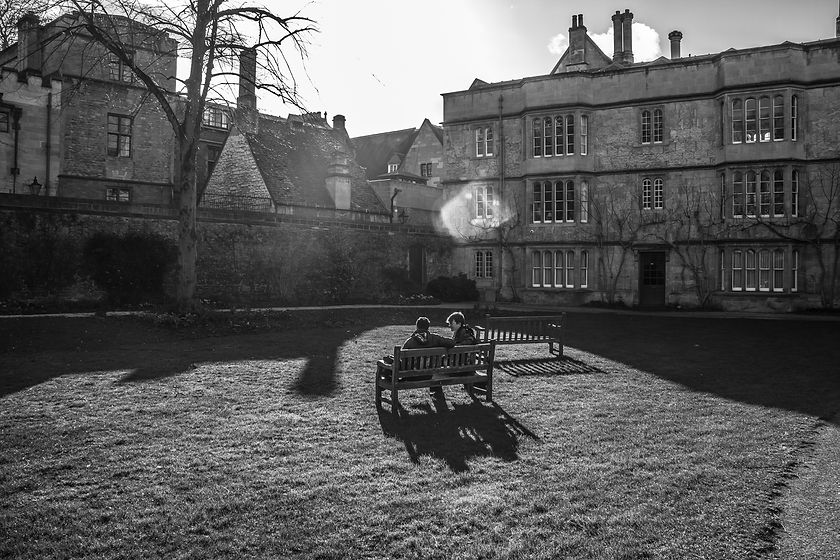
Research Projects & Collaborations

Spanish National Research Council (CSIC)
2024 - Present
MMT24-IEGD-01 Project: Developing Scalable and Standardized AI Tools for Register-Based Administrative Data Collection, Organization, and Analysis
​
Part of the extraordinary grants for Attracting Talent in Digital Skills for CSIC researchers.
​
This Momentum project represents an exciting new chapter in my research career, offering the opportunity to work at the intersection of Artificial Intelligence (AI), Demographics, and Data Science. This project focuses on developing scalable AI models that leverage deep learning techniques, such as convolutional neural networks (CNNs) and recurrent neural networks (RNNs), to extract handwritten text from digitized images and apply these advancements to data linking and transformation. Additionally, the project aims to standardize AI-driven Optical Character Recognition (OCR) processes, integrating state-of-the-art OCR libraries with custom models to improve accuracy and adaptability. A core component of this initiative is the creation of a comprehensive dataset to train and validate AI models, as well as the establishment of a modular and scalable infrastructure for data collection, organization, and analysis.
​
By participating in this project, I expect to gain deeper expertise in AI-driven text recognition, data standardization, and machine learning applications in administrative data systems. This endeavor aligns perfectly with my goal of contributing to technological advancements that enhance the efficiency and accessibility of large-scale demographic and administrative datasets. Embarking on the MMT24-IEGD-01 project is both an exciting challenge and a strategic step in my professional development. One of the key reasons I was drawn to this project is the opportunity to work at the cutting edge of AI applications in social sciences. The fusion of deep learning techniques with historical and administrative records presents a fascinating challenge, one that has the potential to revolutionize the way we digitize and analyze large-scale data sources. At this early stage, I anticipate that this project will provide me with a hands-on, in-depth understanding of scalable AI systems, particularly in their ability to process unstructured data like handwritten records. I am particularly eager to explore how OCR models can be optimized and standardized for different types of historical and demographic records, ensuring not only high accuracy but also adaptability to diverse datasets. Additionally, I see this as an opportunity to develop my skills in building robust AI infrastructures, learning how to create scalable and modular frameworks that ensure long-term usability and interoperability. Beyond the technical aspects, I am also looking forward to collaborating with experts across disciplines—from AI specialists to social scientists—to better understand how advanced computational tools can be integrated into real-world research in demographics and administrative data analysis. I expect that this interdisciplinary approach will challenge me to think critically about the ethical and practical implications of automating data extraction and interpretation, especially when dealing with historical and governmental records. As I move forward in this project, I hope to gain not just technical expertise, but also a broader perspective on the role of AI in social sciences. I am particularly interested in how these advancements can democratize access to valuable datasets, streamline historical research methodologies, and improve the efficiency and accuracy of administrative data processing. This project is more than just an opportunity to refine my technical skills—it is a chance to contribute to the future of AI-driven research in population studies, making complex data more accessible, interpretable, and useful for policymakers, historians, and researchers alike.
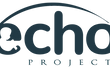

Spanish National Research Council (CSIC)
2019 - 2022
ECHO Project: Early Conditions, Delayed Adult Effects, and Morbidity, Disability, and Mortality in Modern Human Populations
​
Funded by the European Research Council (ERC) under the European Union’s Horizon 2020 research and innovation programme with grant agreement No 788582.
​
The ECHO Project focused on understanding how early life conditions influence adult health outcomes, including morbidity, disability, and mortality. My contributions to this project revolved around the compilation and organization of mortality and morbidity databases, and linking them with spatial determinants. I was involved in analyzing mortality and morbidity data with spatial factors at the community or municipality level, incorporating data visualization techniques to present the findings. Additionally, I helped produce databases resulting from the linkage of multiple information sources, such as censuses, vital statistics, and clinical records. A significant part of the project involved analyzing databanks containing genetic and epigenetic information, including genome-wide association studies (GWAS) and epigenetic clocks, contributing to a deeper understanding of the genetic and environmental factors that affect health outcomes.
This project allowed me to explore complex datasets, enhancing my skills in data linkage, statistical analysis, and genetic epidemiology, while also fostering a deeper understanding of how early life conditions can shape long-term health trajectories. Working on the ECHO Project was a pivotal experience that significantly expanded my skill set in genetic epidemiology, data analysis, and spatial statistics. The compilation and organization of mortality and morbidity databases, along with linking them to spatial determinants, provided a unique challenge in data integration. I developed a more systematic approach to organizing large, heterogeneous datasets and realized the importance of data accuracy and quality control in making meaningful connections across multiple sources. This work reinforced my belief in the need for thorough data cleaning and validation to ensure the robustness of research findings. Analyzing the data with spatial determinants at the community level revealed the strong influence of geography on public health. I learned to appreciate the complexity of health outcomes and how environmental factors can shape mortality and morbidity rates, which is critical for informing public health interventions. Additionally, data visualization became an essential tool in effectively communicating complex trends and patterns, a skill I now prioritize in any project that involves large datasets. The analysis of genetic and epigenetic data, particularly through genome-wide association studies and epigenetic clocks, pushed me to dive deeper into the intersection of genetics and environmental factors in determining health outcomes. It was eye-opening to explore how early life experiences can manifest in later health, and I gained a greater appreciation for the multifaceted nature of public health research. In retrospect, the ECHO Project significantly broadened my perspective on the interplay between genetics, environment, and health. It has also instilled a strong sense of interdisciplinary collaboration, as integrating information from various fields such as genetics, epidemiology, and spatial sciences is critical to understanding and addressing public health challenges. This project has been a defining point in my career, reaffirming my passion for research that connects genetic data with social and environmental factors to improve health outcomes on a population level.

ESRI Spain
2016 - 2019
LongPop Project: Methodologies and Data Mining for Big Data in Longitudinal Population and Epidemiological Registers. Funded by the European Union’s Horizon 2020 research and innovation programme under the Marie Sklodowska-Curie grant agreement No 676060.
The LongPop project provided me with a unique opportunity to work at the intersection of Big Data, epidemiology, and population health research. My work involved the development of a Web Portal for Health and Population in Spain and a Catalogue of Health Resources in Spain, where I enhanced my skills in data integration, system design, and user-centric web development. I explored R functionality within ArcGIS and created custom geoprocessing tools, applying geospatial data analysis to large epidemiological datasets. Additionally, I participated in teaching courses such as the R-ArcGIS Bridge and Up and Running with R, and presented at Esri España national conferences.
This project allowed me to gain significant experience in both technical and pedagogical areas, deepening my understanding of how data science can contribute to public health research. The LongPop project profoundly shaped my professional journey, offering a deep dive into Big Data analytics within the context of public health. My involvement in designing the Web Portal for Health and Population in Spain emphasized the importance of data accessibility and user experience in delivering valuable insights to researchers and policymakers. Working on these tasks sharpened my problem-solving skills, making me more attentive to the balance between technical complexity and practical usability. Exploring the integration of R and ArcGIS pushed my skills in spatial data analysis and taught me the significance of customizing tools for specific research needs. This experience underlined the necessity of combining different data science tools and geospatial techniques to derive meaningful insights from vast, complex datasets. It also highlighted the need for continuous learning and adaptation as new technologies emerge. Furthermore, teaching R-ArcGIS Bridge and presenting at Esri España provided me with valuable insight into the importance of clear communication. Explaining complex concepts to students and peers helped me refine my ability to convey technical information in a comprehensible and engaging manner. These experiences have not only strengthened my research skills but have also deepened my commitment to making data-driven decisions in public health and demographic research.
Research stays
University of Edinburgh, Centre for Research on Environment Society and Health
2017, 2018
During my research stay at the University of Edinburgh, I explored innovative methods in digital epidemiology and historical environmental reconstruction, applying text mining, machine learning, and GIS techniques to analyze health-related behaviors and environments. One major focus was examining the use of Twitter in health research, investigating urban socio-ecological interactions, risky behavioral patterns, and mental health discourse using natural language processing (NLP) and machine learning. Additionally, I contributed to reconstructing historical health environments by analyzing georeferenced text from historic maps, integrating NLP and GIS modeling strategies to study environmental and social conditions over the 20th century as part of the GB1900 gazetteer crowdsourcing project.
This experience not only broadened my expertise in social media analytics and historical GIS but also laid the foundation for an ongoing collaboration with the University of Edinburgh, where I continue to explore new research directions at the intersection of geospatial analysis, AI, and public health. My time at the University of Edinburgh was a transformative experience that significantly shaped my research interests and methodological approach. Engaging with digital traces of human behavior, such as social media data, allowed me to witness firsthand how emerging technologies can provide valuable insights into public health and urban dynamics. The challenge of extracting meaningful signals from Twitter discourse on mental health reinforced the complexities of text mining, making me more aware of the nuances in sentiment analysis, bias in datasets, and the ethical considerations of using digital data for research. Beyond contemporary health studies, my work on reconstructing historical health-related environments deepened my appreciation for longitudinal spatial analysis. The integration of NLP with GIS to analyze text from historic maps was particularly insightful, as it demonstrated how modern computational techniques can be leveraged to decode the past and understand long-term health and environmental trends. This aspect of the project reinforced my belief in the value of interdisciplinary research, where techniques from data science, history, and geography converge to generate new knowledge. What made this research stay especially impactful was the collaborative environment at the Centre for Research on Environment, Society, and Health. Working with experts in geospatial epidemiology, digital health, and data science provided me with new perspectives on methodological integration and the importance of translating research into actionable insights. The relationships and collaborations I built during this period have continued beyond the LongPop project, allowing me to further develop my work in spatial data analysis and AI-driven public health research. Looking back, this experience reaffirmed my passion for bridging computational methods with health and environmental research. It not only equipped me with advanced technical skills but also enhanced my ability to approach complex problems with a multidisciplinary mindset. As I continue to collaborate with the University of Edinburgh, I am excited to further expand my research in digital epidemiology, historical spatial analysis, and AI applications in public health.